With data architecture and data storage systems becoming a critical part of digital transformation and AI journeys, what are some of the best practices regional enterprises should adopt for data and storage?
Large global companies are struggling with the challenge of using their data to help them run their businesses better. With more data than ever before and new data sources popping up daily, organisations have critical information dispersed and siloed that they cannot trust or derive accurate insights from. Executives from Cloudera, Cloud Box Technologies, NetApp, Pure Storage, respond on this question.
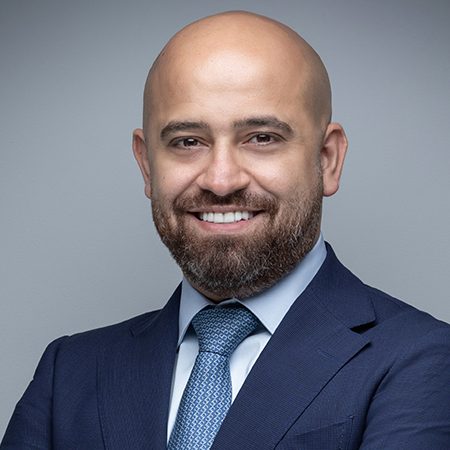
Ahmad Shakora, Group Vice President Emerging Markets, Cloudera
Hybrid is the new de facto standard, with 76% of organisations in the Middle East storing both on-premises, private cloud and the public cloud, according to a Cloudera study. However, companies find it difficult to fully extract value from their data assets across a mosaic of hybrid and multi-cloud environments.
Almost three-quarters, 74% of respondents agree that having data sitting across different cloud and on-premises environments makes it complex to extract value from all the data in their organisation. Solving these challenges is essential. Companies need to take back control of their data, analytics, and AI with a unified platform built on openness.
A true hybrid data platform enables enterprises to analyse and bring GenAI models to their data wherever it lives, hybrid, multi-cloud, or on-premises.
The trustworthiness of AI starts with being able to trust the data the models are trained on. As AI regulation remains in flux, customers must stay compliant by knowing their greatest asset, data in their models is secure while supporting continuous innovation for whatever comes next.
Companies need to generate and operate their enterprise AI models where their most private and secure data resides – essentially allowing enterprises to bring their AI models to the data, not their data to the models.
Regarding data storage, many organisations realise that certain tasks are more costly in the cloud than expected. They now prioritise choosing the best environment for specific workloads. Decisions between cloud-native deployment and on-premises hosting should be data-driven.
Workload analytics help assess performance before deciding. Stable, predictable tasks often cost less on-premises, while variable, customer-facing services benefit from the cloud’s elasticity.
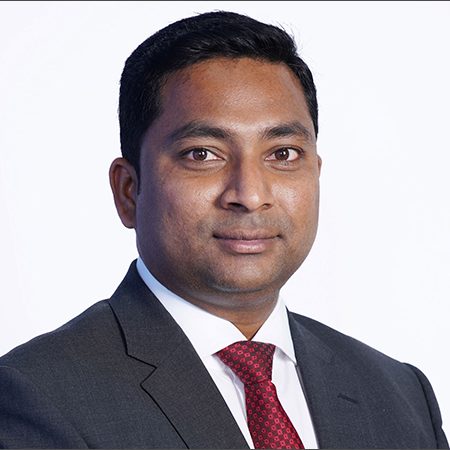
Avinash Gujje, Practice Head at Cloud Box Technologies
Enterprises are undergoing a paradigm shift due to the ongoing wave of digital transformation. As data has become a driving force, enterprises are recognising it as a competitive advantage to gain a strategic edge over competitors.
Robust data architecture and data storage systems play a pivotal role in optimising current operations while fortifying future endeavours to ensure resilience and navigate through ever-changing market dynamics. For that reason, organisations must adhere to certain best practices to stay ahead.
Cloud storage solutions have empowered organisations to accommodate exponential data growth without falling into the perils of incurring huge costs or compromising performance. Modern cloud storage solutions offer impeccable resilience against such storage requirements.
They dynamically adjust both storage capacities and processing required based on current demands. It ensures minimal upfront costs and optimised resource utilisation. Moreover, the advent of AI in cloud data storage allows enterprises to refine their parameters to gain desired output.
Another best practice is setting up data backups and recovery systems. Modern data storage solutions offer impressive backup and recovery strategies, enabling organisations to create redundant copies of data spread geographically across various data centres. This prevents any data loss due to system failure, natural calamities, or cyberattacks.
Organisations opting for digital transformation must adhere to regulatory standards and cybersecurity measures. It ensures proper risk mitigation techniques are in place in the event of data breaches. With a data governance framework, organisations can specify data policies, accountability, and responsibility mechanisms that ensure relevant parties take necessary steps to comply with standards and regulations.
As data has become an integral driving force behind innovation and unparalleled growth for companies, leaning into AI-based data-driven decision-making ensures organisations use their data assets to their full potential.
AI-based data analytics provide insights into customer journeys and preferences allowing in-house departments to refine their operations to ensure higher customer satisfaction. Data analytics can assist in identifying shortcomings, eliminating bottlenecks, and driving higher operational efficiencies.
Unlike a few years ago, embarking on a digital transformation journey has become a differentiating factor propelling enterprises into the digital age. Modern data architecture and storage systems ensure sustainable and scalable growth, data resilience, and integrity, as well as offer analytics, giving companies a strategic advantage to navigate through the volatile market dynamics.
These are some of the best practices that can unlock floodgates of opportunities propelling regional enterprises to the next level, especially in a hyper-saturated and competitive market such as the Middle East.
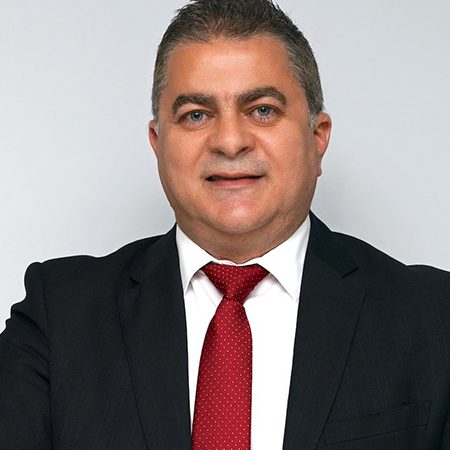
Walid Issa, Senior Manager, Pre-Sales and Solutions Engineering, MENA and East Europe, NetApp
For regional enterprises embarking on their digital transformation and AI journey, adopting best practices for data architecture and storage systems is crucial to ensure efficiency, scalability and resilience.
AI will create a lot of potentials that will accelerate innovation, revolutionise operations, and deliver superior solutions that will impact the way we do business. However, there will be challenges as the foundation of AI information which is data, and data is everywhere, at the edge, on premises, inside apps, and in multiple public clouds.
Therefore many enterprises end up dealing with Silos and bottlenecks that hold data back and hence causes challenges to manage and use this various data and data types. In addition, this Data is constantly under threat and therefore enterprises need robust data management to keep models, data, and usage safe.
Artificial intelligence demands data that relies on an intelligent data infrastructure. Regional enterprises need to take a unified approach to data and build an intelligent data infrastructure that breaks down these silos. They need to build their AI data infrastructure and solutions to enable performance, productivity, and protection for their data and their AI anywhere.
And with more enterprises considering Generative AI, and the associated extreme scale and massive datasets involved with large language models, LLMs, it is crucial to architect a robust AI infrastructure that takes advantage of data storage features on-premises, hybrid and multicloud deployment options and reduce risks associated with data mobility, data protection and governance before companies can design AI solutions.
They would need a strong foundation which is the right intelligent data infrastructure to meet the complexities created by rapid data growth, data mobility, multi-cloud management, and the adoption of AI without compromising on the ability to expand while maintaining cost-efficiency, data governance and ethical AI practices in control.
At NetApp can help regional enterprises build an intelligent data infrastructure that shatters silos, optimizes AI and GenAI, and delivers agility for a rapidly changing landscape. Our unified approach to infrastructure and data management enables performance, productivity, and protection everywhere with turnkey solutions that accelerate time to results.
All of that is coupled with deep technological partnerships with AI leaders like NVIDIA, where NetApp is delivering certified, AI turnkey solutions. NetApp’s family of AI-focused products continues to evolve to meet customer needs, and we are excited to share and partner with regional enterprises on this journey.
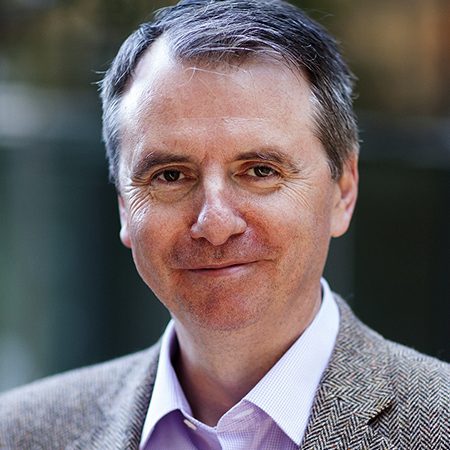
Alex McMullan, CTO International, Pure Storage
To understand the challenges that AI presents from a data storage perspective, we need to look at its foundations. Any machine learning capability requires a training data set. In the case of generative AI, the data sets need to be large and complex, including different types of data.
Generative AI relies on complex models, and the algorithms on which it is based can include a large number of parameters that it is tasked with learning. The greater the number of features, size and variability of the anticipated output, the greater the level of data batch size combined with the number of epochs in the training runs before inference can begin.
Because data volumes are increasing exponentially, it is more important than ever that organisations use the densest, most efficient data storage possible, to limit sprawling data centre footprints, and the spiralling power and cooling costs that go with them. This presents another challenge that is beginning to surface as a significant issue: the implications massively scaled-up storage requirements have for being able to achieve net zero carbon targets by 2030-2040.
Some technology vendors are already addressing sustainability in their product design. For example, all-flash storage solutions are considerably more efficient than their spinning disk, HDD counterparts. Some vendors are even going beyond off the shelf SSDs, creating their own flash modules.
As well as being more sustainable than HDD, it is also a fact that flash storage is much better suited to running AI projects. This is because the key to results is connecting AI models or AI powered applications to data.
To do this successfully requires large and varied data types, streaming bandwidth for training jobs, write performance for checkpointing, and checkpoint restores, random read performance for inference and crucially it all needs to be 24×7 reliable and easily accessible, across silos and applications. This set of characteristics is not possible with HDD based storage underpinning your operations, all-flash is needed.
Data centres are now facing a secondary but equally important challenge that will be exacerbated by the continued rise of AI and ML. That is water consumption, which is set to become an even bigger problem.
As AI and ML continue to rapidly evolve, the focus will increase on data security, to ensure that rogue or adversarial inputs cannot change the output, model repeatability, using techniques like Shapley values to gain a better understanding of how inputs alter the model and stronger ethics, to ensure this powerful technology is used to actually benefit humanity.
Click below to share this article