Gartner research shows that data literacy will become a necessary driver of business value and success will be linked to how well employees can speak data, making informed real-time decisions for the business, avoiding being surpassed by competitors, explains Vishal Soni at Alteryx.
AI is everywhere. It is in your television, it is in your online shopping basket, and it is in your car tyres. According to Accenture, AI could contribute approximately $215 billion and $182 billion as annual gross value add, GVA to the economies of Saudi Arabia and UAE respectively, by 2035. It is one of the most powerful tools that businesses can use to make fast and accurate decisions from their data. It is also one of the most regularly misunderstood technologies available.
AI to a technology specialist means a system capable of taking information and delivering insights from existing data, this can be as simple as a decision tree, or as complex as a compute-heavy heuristic model. To a non-technical person, however, AI means a supercomputer in a high-tech laboratory.
Call that same AI system an algorithm or a recommendation engine, however and eyes light up, understanding happens. It is how Netflix takes what you watch and recommends what you might like to watch next, taking data from past events and extrapolating it to recommend a future action. What happens when you tell a non-technical employee to start using AI in their day-to-day?
Jargon to block
This is the challenge that businesses are facing. The tools they need to stay competitive and mitigate market disruption through better use of data are ready to use but remain hidden behind layers of misunderstanding. With shifting global economic landscapes and a greater need to process data to deliver insights, the jargon issue has progressed from a minor annoyance to a critical business blocker.
By 2023, Gartner research shows that data literacy will become an explicit and necessary driver of business value. This means that success is linked to how well employees can speak data. This data-driven insight generation can be the difference between making informed real-time decisions for the business and the risk of being surpassed by competitors who have prioritised digital skills such as data literacy.
Where data science was once a highly siloed and exclusive discipline, history shows that subject matter experts are in the best position to deliver benefits through data insights. AI and Machine Learning are two of the most powerful of the insight-generating tools available.
Despite this, business leaders report that expanding the remit of these knowledge workers can be challenging in change management. Further, the systems they are expected to use can often be improperly delivered.
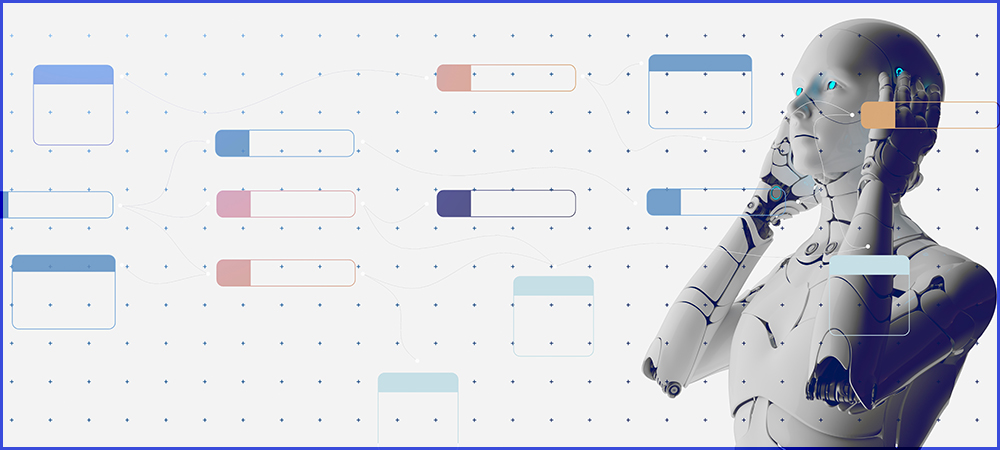
Knowledge and data
Businesses now desperately need these upskilled experts who can take their own data and answer their own questions. With vast volumes of historic data, and huge potential benefit to delivering insights at speed, the medical sector is primed and ready for the benefit of analytics.
In recent years, the MENA region has made remarkable progress in integrating artificial intelligence, AI into its healthcare sector to help doctors use their cloud data to work faster and more effectively. This forward-thinking approach is evident through the introduction of the Patient Risk Profile functionality within Malaffi, the region’s groundbreaking Health Information Exchange, HIE platform.
The risk management solution employs advanced AI technologies and machine learning algorithms, effectively leveraging demographic, and clinical data from the population, to deliver valuable insights and predictive models. However, what doctors and healthcare experts know to look for in a patient’s medical profile is not always the same thing a data scientist will be able to build into an AI model.
The understanding gap between knowledge expert and data scientist actually works both ways.
- Data science teams can create models without the subject matter expertise needed for that model to perform as intended.
- The knowledge workers may get blocked from supporting data science teams due to jargon-heavy inaccessible technologies.
With enough collaboration bolstered by improvements in data literacy and more immediately understandable technologies, we have a direct experience-led cartographer between the medical data and the data scientist, the upskilled domain expert.
Tools in the right hands
The best examples of turning data into action have frequently come from subject matter experts. For example, a logistics company trying to choose the optimal route for a delivery should ask their driver, not a member of their data science team.
While the data scientist is aware of macro trends, the driver knows the micro changes that may otherwise be hidden. When we ask the in-department experts, we get answers with vital context and background information, details that are only ever visible after years of direct experience.
Companies looking to overcome the shortage of data skills must focus on empowering their existing workers, breaking down the jargon factor and positioning these productivity-boosting tools as simply as possible.
In the exponential era, defined by exponential growth of data created, of skills shortages, and of net new disruption on a daily basis, learning how to deliver more with less is mission critical for business success.
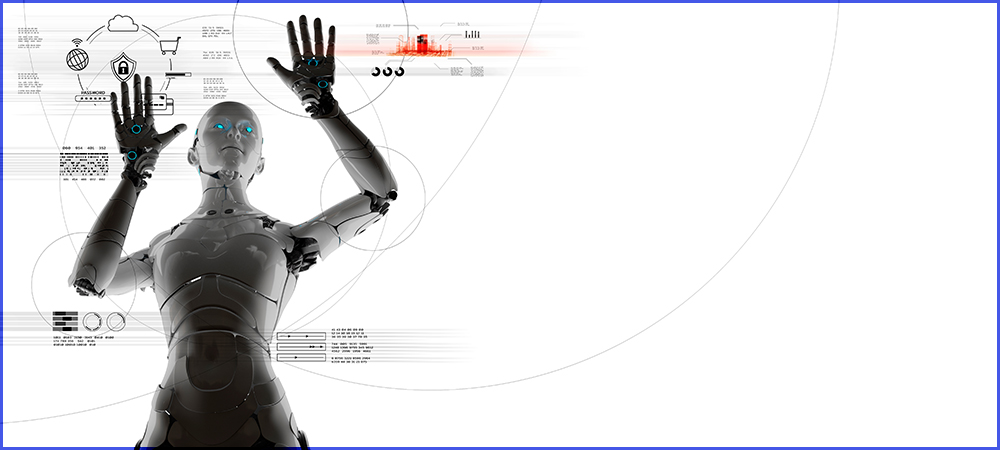