Lim Hsin Yin, Managing Director of Singapore, SAS Institute, says that while many organisations are already leveraging generative AI to their advantage, it is crucial not to overlook the importance of deploying it in a responsible, ethical and safe manner.
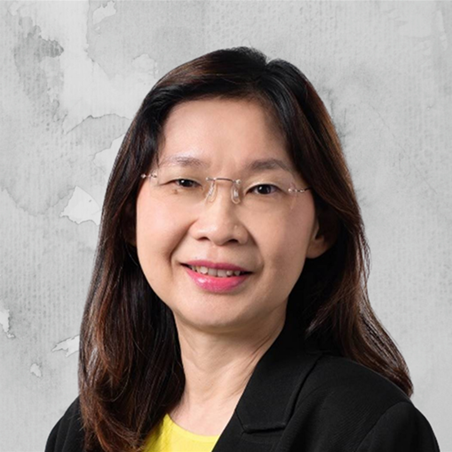
Decades ago, AI was depicted in the media as either a fantastic transformer of worlds or a dystopian entity.
With generative AI being the talk of the town, many are talking about its groundbreaking benefits and potential use cases.
For instance, generative AI can enable financial institutions to tailor investment strategies to individual client preferences. In healthcare, it could help providers automate the transcription of clinician-patient interactions.
Meanwhile, manufacturers could utilise it to conduct predictive maintenance on assembly lines. This demonstrates the ability of generative AI to go through massive amounts of data to analyse and derive insights from, gain human-like recognition of natural language, and even replicate and automate human behaviours.
But many are also talking about the challenges of Artificial Intelligence, both actual and potential, as its development poses questions of existing regulatory frameworks. Failure to do so could lead to reputational damage, a loss of public confidence in AI, and potentially burdensome government regulations in response.
As the responsibility over ethical AI use is as much one for businesses as it is for governments, the former should proactively address these challenges by integrating principles of ethical AI into their generative AI projects from Day One.
One of the areas of concern regarding generative AI laid out by Singapore’s National AI Strategy (NAIS 2.0) is the current inadequate levels of transparency regarding large language models (LLMs). It is critical in light of questions about the existence of biases that have been built into the models and affect the validity, credibility and even legality of their outputs.
Similar to children who take up after their parents, AI models can unintentionally absorb patterns from the data they are trained on due, in part, to insufficient awareness among data scientists regarding historical and societal biases that may be present in the data.
This lack of awareness can have far-reaching consequences in various fields. For example, in healthcare, biases in data or algorithms can negatively impact patient care and resource allocation, while those in human resources can affect recruitment, evaluation and decision-making processes.
It is crucial to address biases and actively work towards creating more inclusive AI systems. SAS has outlined six core principles to guide responsible innovation, including the use of AI. These are human centricity, transparency, inclusivity, privacy and security, robustness and accountability.
To foster accountability in using AI, organisations must recognise that it is a shared responsibility of all people and entities involved in an AI system.
One way to encourage accountability is by implementing clear decision workflows that assign ownership and add transparency to the AI system. These allow users to create, approve, annotate, deploy and audit decisioning processes – while also maintaining a record of who was involved.
An AI system designed with accountability in mind should also include mechanisms for customer feedback, error remediation and correction. Observing and auditing AI flows will help organisations identify and address any issues as soon as possible, enabling them to proactively mitigate concerns before they escalate.
Implementing feedback loops also allows AI systems to learn and adjust their behaviour based on user input.
To enable the active monitoring of various aspects of AI system performance, such as data drifts, concept drifts and out-of-bounds values, organisations require platform capabilities like bias detection, explainability, decision auditability, model monitoring and other governance and accountability measures.
Organisations should strive to involve more non-technical roles in the AI conversation. It is not enough for technologists to dictate the AI agenda to be solely determined by technologists when there are implications for justice, well-being and equity.
Non-technical domain experts are better suited to consider these implications and uncover risks and opportunities.
By involving a diverse range of perspectives, organisations can put themselves in the best possible position to recognise and respond to AI ethical risks at the point of transaction. This inclusivity ensures that potential ethical issues are addressed appropriately and allows for a more comprehensive approach to managing AI risks.
While many organisations are already leveraging generative AI to their advantage, it is crucial not to overlook the importance of deploying it in a responsible, ethical and safe manner. Proactively addressing the potential negative impacts of unethical AI use is vital for the long-term success and reputation of an organisation. By prioritising responsible deployment and usage, organisations can mitigate risks and ensure that the benefits of generative AI are maximised while minimising harm.
Click below to share this article