While AI-ML has the potential to revolutionise African industries and transform decision-making, it is important to remain vigilant explains Gary Allemann at Master Data Management.
Over the past few months, there has been a lot of hype about ChatGPT and its wonders. Make no mistake, ChatGPT is popularising Artificial Intelligence to the masses and certainly can add tremendous value for selected use cases.
However, sometimes AI tools’ logic can be flawed, alongside its basic arithmetic. Now imagine this was calculating your risk profile, to see whether you qualified for a lower interest rate, or even to buy your first house. Does this mean that all AI is bad? No, but it does illustrate the importance of AI governance and data integrity.
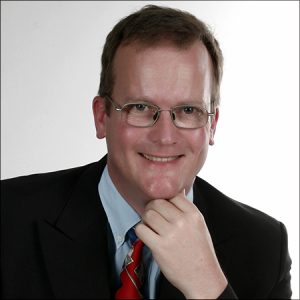
We cannot simply assume that AI or Machine Learning will work with any dataset and any use case, we need to test and manage changes to both the data and the model to ensure the integrity of results.
AI Governance
AI governance refers to the set of principles, policies, and regulations that guide the development, deployment, and use of AI systems. The aim of AI governance is to ensure that AI is developed and used in a way that is safe, transparent, ethical, and accountable.
We need AI governance for several reasons. First, AI is rapidly advancing and has the potential to impact many aspects of society, including employment, healthcare, and security. Therefore, it is crucial to ensure that AI is developed and used in a responsible and ethical manner.
Second, AI systems can be biased or make decisions that are unfair or discriminatory, which can have serious consequences for individuals and groups. AI governance can help to mitigate these risks and ensure that AI is used in a fair and equitable way. Third, AI governance can help to build trust and confidence in AI systems, which is essential for their widespread adoption and use.
Key components of AI governance include:
Standards and guidelines
AI governance establishes standards and guidelines for the development and deployment of AI systems. These standards and guidelines help ensure that AI systems are developed and used in a way that aligns with ethical, legal, and social norms.
Oversight and accountability
AI governance ensures that individuals and organisations are accountable for the development and use of AI systems. Oversight mechanisms, such as audits and assessments, are put in place to ensure that AI systems are transparent and that their outcomes are explainable.
Risk assessment
AI governance involves assessing the risks associated with the development and use of AI systems. This includes identifying potential risks, such as bias, discrimination, and privacy violations, and implementing measures to mitigate these risks.
Collaboration and engagement
AI governance involves collaboration and engagement with stakeholders, including industry, government, civil society, and the public. This collaboration ensures that the development and use of AI systems are aligned with the needs and values of society.
Overall, AI governance is necessary to ensure that AI is developed and used in a way that is beneficial to society and aligned with our values and ethical principles.
Data governance
Data governance and AI governance are related concepts, but they are distinct in their focus and scope.
Data governance refers to the overall management of the availability, usability, integrity, and security of data used within an organisation. Data governance includes establishing policies, standards, and procedures for data management, and ensuring that data is collected, stored, and used in a way that complies with legal and ethical requirements.
On the other hand, AI governance refers to establishing policies and standards as well as managing of the development, deployment, and application of AI systems in order to guarantee their safety, reliability, and objectivity, as well as ensuring that AI systems are used in a way that complies with legal and ethical requirements.
In other words, while data governance is focused on managing data as an asset, AI governance is focused on managing the development and deployment of AI systems as a technology. AI governance builds on the foundation of data governance but extends it to include the unique challenges and risks associated with AI, such as algorithmic bias, explainability, and accountability.
AI Governance can form part of your data governance framework, which can benefit from investments in data catalogues and other platforms that assist data stewardship.
Accurate results
Ensuring that AI delivers accurate and unbiased results is a complex and ongoing challenge. However, here are a few key approaches that can help:
Use high-quality, diverse data
The data used to train AI algorithms should be representative of the real-world situations in which the AI will be used. Diverse data sets, which include different types of people and experiences, can help prevent biased outcomes.
Regularly audit and test AI models
It is important to monitor and evaluate AI models regularly to ensure they are delivering accurate and unbiased results. Testing the model with new data, verifying the results, and comparing them to benchmarks can help identify and correct issues.
Involve a diverse team
Building an AI team with diverse backgrounds and perspectives can help identify biases and ensure that the AI models are designed to be fair and unbiased.
Ensure transparency and accountability
It is important to be transparent about the data and methods used to develop AI models and to have clear guidelines and processes for handling issues that arise.
Regularly update models
AI models need to be regularly updated to ensure they continue to deliver accurate and unbiased results. This can include updating the data used to train the model, refining the algorithms, and testing the model in new situations.
Overall, ensuring that AI delivers accurate and unbiased results requires ongoing effort and attention. It’s important to approach the development and use of AI with a critical and cautious mindset, while also recognising its potential to bring significant benefits to society.
Data quality
Data quality plays a crucial role in ensuring accurate results from AI systems. The quality of the data used to train and test AI models directly affects the accuracy and reliability of the results produced by the model.
Here are some reasons why data quality is important for AI:
Garbage data
If the data used to train an AI model is of poor quality or contains errors, the resulting model will produce inaccurate or biased results. This is commonly referred to as the “Garbage In, Garbage Out” principle, which highlights the fact that the output of an AI model is only as good as the input data.
Bias
Biased data can result in biased models. If the data used to train an AI model is biased, the resulting model will likely produce biased results. This is particularly concerning in applications where fairness and non-discrimination are important, such as hiring or lending decisions.
Results
Data quality directly affects the accuracy and reliability of results produced by AI models. Clean, accurate, and high-quality data leads to more accurate and reliable results from AI models.
To ensure accurate results from AI, it is important to have a robust data quality management process in place. This process should include data cleaning, validation, and monitoring to ensure that the data used to train and test AI models is of high quality and free from bias.
Additionally, it is important to continually evaluate the data quality throughout the AI system’s lifecycle and to incorporate feedback from users to improve the accuracy and reliability of the results produced by the system.
While AI and machine learning models have the potential to revolutionise industries and transform the way we approach decision-making, it is important to remain vigilant and ensure that these models, and the data that feeds them, are constantly monitored, and updated to deliver reliable results.
In AI, the accuracy of the results produced by models depends heavily on the quality of the data being used to train them. Data that is inaccurate, incomplete, or biased can lead to incorrect or biased results, which can have serious consequences in areas such as healthcare, finance, and law enforcement.
Even with the best training data, it is important to note that AI and machine learning models can yield inconsistent results if the real-world data they are interpreting varies significantly from the data used during their initial training.
This discrepancy can occur when the training data does not accurately reflect the complexities and variations present in the real-world data, which can lead to biased and unreliable results. Additionally, external factors such as changes in the environment, user behaviour, and technology can also contribute to this disparity.
Data observability helps ensure that the data being used by AI models is of consistent quality and remains suitable for the intended purpose. It involves tracking, monitoring, and analysing data movements to identify significant shifts in the state of the data used to feed AI and ML models.
In some instances, these issues may be the result of a failure in a data pipeline, which can be corrected by the operations team. In other cases, data observability may indicate long terms shifts in data that require the AI model to be retrained, using new, more representative training data.
In addition to maintaining the accuracy of AI results, data observability provides transparency into the data used by AI models, which is increasingly important as organisations face growing scrutiny over the use of AI and its potential impacts on society.
Overall, data observability plays a crucial role in ensuring that AI produces accurate and reliable results over time, and helps organisations build trust in the use of AI in their operations.
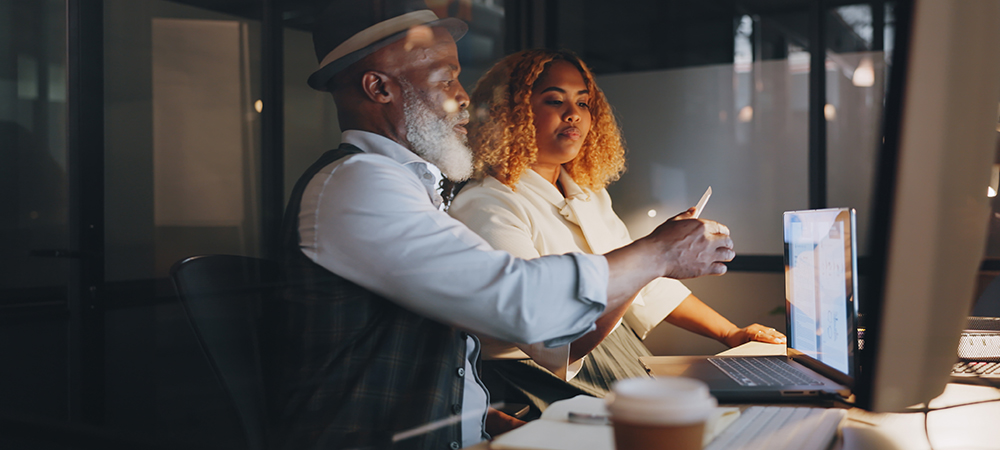