A flurry of AI models, generative applications, engineering tools have been released in recent months, indicative of the competitive jostling characteristic of high-stakes, early-stage markets, but are also confusing African CIOs with an array of options, says Arun Chandrasekaran at Gartner.
The popularity of ChatGPT has sparked interest in the adoption of generative Artificial Intelligence, AI across industries. A flurry of AI foundation models, generative AI applications and AI engineering tools have been released in recent months.
While these rapid developments indicate the competitive jostling that is characteristic of most high-stakes, early-stage markets, they also provide enterprise IT leaders with a confusing array of options, making it difficult to choose the right deployment approach for generative AI.
Gartner predicts that by 2026, more than 70% of independent software vendors, ISVs will have embedded, generative AI capabilities in their enterprise applications, which is a major increase from fewer than 1% today.
To make informed decisions and derive value, Chief Technology Officers, need to understand the various approaches available and outline a decision framework for choosing one over the other.
Consume generative AI
Organisations can directly use commercial applications that have generative AI capabilities embedded in them. It is easier to deploy with low or no fixed costs required to start experimenting with generative AI capabilities. Moreover, easy integration with existing workflow makes this the least-disruptive approach.
However, a strong dependence on the application provider’s security and data protection controls, can lead to security and data privacy risks for organisations. There are also chances that applications with embedded AI may not be able to deeply understand the context of a conversation or task, leading to less-accurate or less-relevant responses.
Embed generative AI APIs
Enterprises can build their own applications, integrating generative AI via foundation models APIs. Most closed-source generative AI models, GPT-3, GPT-4, PaLM 2, etc. are available for deployment via cloud APIs. This approach can be further refined by prompt engineering. In prompt engineering, the underlying foundation model is frozen — this provides the ability to use the same model across a variety of use cases.
Additionally, foundation models can perform new tasks with adequate accuracy with a limited number of high-quality samples. This approach has its own benefits, but prompt engineering is a nascent field, where best practices are only emerging, and for which new skills are required.
Extend generative AI models
Retrieval augmented generation, RAG enables enterprises to retrieve data from outside a foundation model, often your internal data and augment the prompts by adding the relevant retrieved data. This will improve the accuracy and quality of model response for domain-specific tasks. Extending the models via a RAG approach can provide an appropriate balance between bringing organisational context into foundation models without the complexity and cost of modifying the underlying models.
However, implementing a RAG approach involves redesigning the technical architecture and workflow to include new technology components. The knowledge about these technology components and the overall architecture is pretty rudimentary in most enterprises. These additional components also carry additional costs.
Extend via fine-tuning
Fine-tuning takes a large, pretrained foundation model as a starting point and further trains it on a new dataset to incorporate additional domain knowledge or improve performance on specific tasks. This often results in custom models that are dedicated to the organisation. This approach can result in improved performance and reduce hallucinations as the models are fine-tuned with organisational data and, or domain-specific data for particular tasks.
But foundation models fine-tuned for specific use cases might lose their ability to be extended to broader use cases. Moreover, the cost of using a fine-tuned model, inference cost can be significant, even if the cost of fine-tuning training is not high.
Build custom foundation models
Organisations could ultimately build their own foundation models from scratch, fully customising them to their own data and business domains. If adequate data governance is in place, then the organisation will have complete control over the training datasets and model parameters.
This can significantly increase use-case alignment and reduce bias. This approach grants greater control to the organisations over the model, however, the cost of training and maintaining a large, generative AI model can be exceedingly high.
Most organisations can deploy some, if not all, of the approaches described above, depending on the use case, technical knowledge, maturity of the organisation, and time-to-market requirements.
When comparing deployment approaches and choosing the one that delivers business value, IT leaders need to be aware of various important factors – total cost of ownership, TCO, integration of organisational and domain knowledge, implementation complexity, model accuracy performance and ability to control security and privacy.
Role of open source
In the realm of generative AI, open-source models are increasingly playing a crucial role in democratising access to AI. Open-source models have the potential to further digital advancement and lower the barrier for experimentation with Generative AI. In addition to making technology more accessible, open-source models foster a sense of collaboration. Nevertheless, as with all things, open-source models have their own advantages and drawbacks.
IT leaders seeking better visibility, control and customisation of generative AI deployments must consider the pros and cons of open-source models outlined below.
Customisability
Open-source models can be customised to meet the needs of organisations as developers have access to the model parameters and source code. This helps enterprises to have a better control over costs, output, and alignment with their use cases.
By owning open-source-model-based products, enterprises can continuously evolve them to meet internal and customer demands. It also makes their applications harder for competition to imitate.
Control over privacy
A key reason for enterprise interest in open-source models is that enterprises can potentially run generative AI models across the environment of their choice, on-premises, cloud or edge, which gives them significant control over data and security risks, for example, no need to send any data to the cloud if it is hosted on-premises.
Community improvement
Using open-source models, enterprises can tap into the power of development communities, which seek to constantly refine these models. Of course, this is dependent on the vibrancy of the community.
Transparency
Open-source models can be more thoroughly inspected and analysed, which could not only boost confidence in their adoption, but also enable enterprises to meet future regulatory requirements more effectively.
Vendor lock-in
The adoption of open-source models can reduce the strength of vendor lock-in. The landscape of generative AI models is rapidly evolving, so open source can provide users with more flexibility to swap models or model providers, with fewer exit barriers.
Longer time to value
The investments in data engineering, tooling integration and infrastructure to train and run these models can be high, particularly for larger models. This represents a significant fixed cost and longer time to value when compared with proprietary alternatives.
Complex model
It will be harder to upgrade and manage the life cycle of open-source models when they come out, particularly if significant customisation was built on top of a given version. Additionally, maintaining consistent performance and quality standards across various model releases can also be challenging.
Varied licensing
There are a variety of licensing models within open source today. This can impose restrictions for the consumer and will require rigorous review from legal teams before adoption. For example, not all open-source models are certified for commercial use.
Skills
It takes dedicated personnel with deep expertise in fine-tuning and model operations to customise and host these models. For most companies, this is a tall order.
Gap
There is currently a gap in terms of accuracy between proprietary LLMs and open-source LLMs models, as measured by different benchmarks. This gap might narrow over time, as new models emerge.
Open-source generative AI models, such as Bloom, Llama 2, and Starcoder have their unique strengths and weaknesses. IT leaders should consider the pros and cons and perform an objective analysis of open-source models on a case-by-case basis. Ultimately, before implementation of these models in production scenarios, a meaningful, unbiased analysis of total cost of ownership, TCO and risk assessments should be conducted.
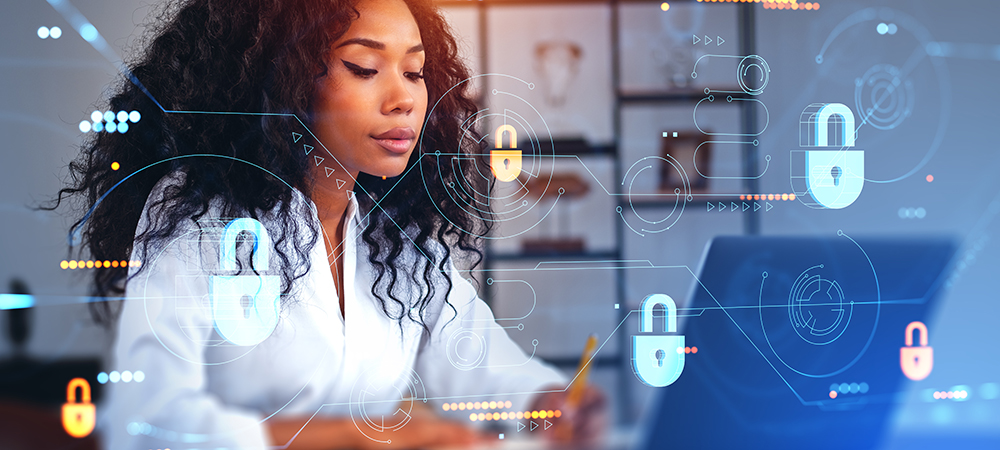